A Different Path Of Predictive Maintenance
Reduce unplanned down time
Get machine condition report in minutes
Save DT implementation cost
Base on unique un-supervised machine learning
Translate Machine Data into Stable & Sustainable Performance
Our Goal
INGSHI focuses on research & development of Dynamic Predictive Analytics algorithms and solutions. We empower our clients with advanced data intelligence to make use of data to improve productivity and quality, and to reduce unplanned downtime and overall cost in R&D, production management and asset management.
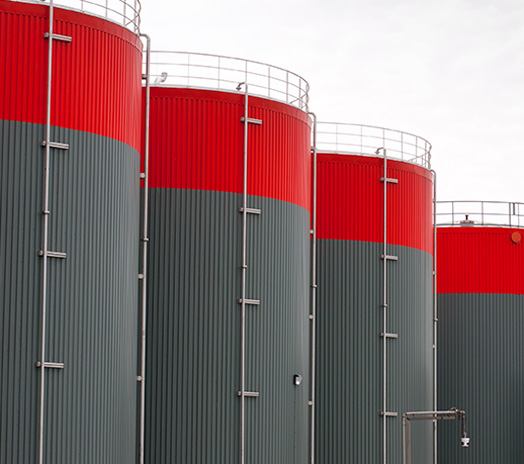
Guaranteed ROI
Our Products
Boost your profitability by surpass others!
Dataneuro is incredibly powerful easy-to-use ready-to-use !
Dataneuro is a technology for machine and process condition prognostics via pattern recognition and modeling of multi-sensor analog data. NOST integrates with AI pattern recognition and unsupervised machine learning mechanism which can carry out pattern mining and analysis for a large amount of multi-dimension analog data with impressive speed and accuracy, and with incremental learning capability.
Reduce Downtime | Improve Sustainability | Precision Maintenance
Dataneuro RT.NET: Machine Condition Prognostics System
Creates machine condition models without lengthy and costly process and applies models to identify omens of machine malfunctions in advance with little human effort.
Modeling with Data Fusion
Based on machine sensor data, to automatically create single condition model for each machine.
Real-time Condition Prognostics
Machine condition assessment based on the condition model and real-time sensor data.
Adaptive Model Optimization
The machine condition model self-optimizes automatically when new condition is captured.
Reduce Downtime | Predictive Maintenance | Reduce maintenance Costs
Dataneuro ME.NET: Machine Condition Assessment System
Based on sensor data collected periodically, the conditon of machine is evaluated continuously, and health trend of the machine is assessed.
MModeling with Data Fusion
Based on machine sensor data, to automatically create single condition model for each machine.
Periodical Condition Assessment
Based on periodical operation data, condition and health trend of the machine is evaluated continuously.
Adaptive Model Optimization
The machine condition model self-optimizes automatically when new condition is captured.
It costs approximately 50% more to repair a failed asset than if the problem had been addressed prior to failure.
Production capacity is lost to as much as 5% every year as a result of unplanned machine down time.
Inrease Analysis Efficiency | Improve Quality | Optimize Process
Dataneuro FC.NET: Production KPI Forecast & Parameter Optimization
Key process parameters, like the quality of raw materials, production controls, and machine conditions, have significant impacts on the production process and the quality of products. Typically, a particular value combination of key process parameters will lead to better or expected product quality. It’s valuable to find out the optimal combinations of process parameters through data mining.
FC.NET creates a library of patterns (combination of process parameters) based on process data via unsupervised incremental machine learning, which it’s easier for engineers to get the correlation between optimal process parameters related to the optimal or expected product quality.
Production KPI Forecast Model
Build correlative forecast model with production KPI and process parameterd.
Process Parameter Optimization
Get optimal process parameters for specified production condition.
Adaptive Model Optimization
Forecast and optimization models will upgrade according to real production conditions.
Efficient Condition Modeling Early Anormaly Detection Quantized Health Trend Assessment Dynamic operation KPI forecast
Case Study
Machine Condition Assessment & Abnormal Analysis
A serious malfunction of the asset happened. A fault alarm was triggered from the monitoring system ONLY a few minutes ahead. The client wanted to know if there was an omen before the malfunction. The client provided historical sensor data in 6 weeks around the machine malfunction for analysis.
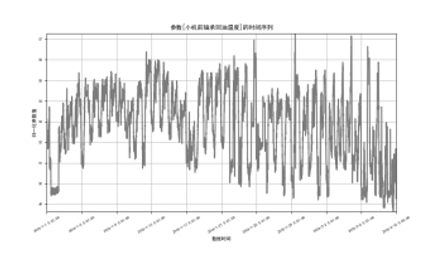
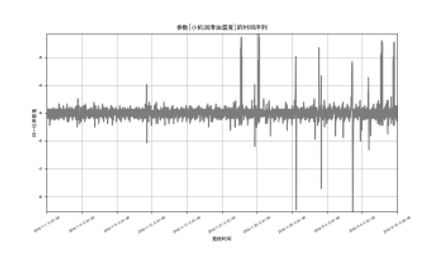
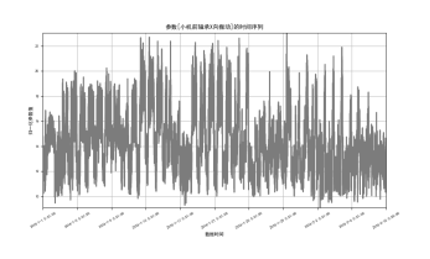
35 sensors, including vibration, flow, temperature, pressure, displacement, etc.
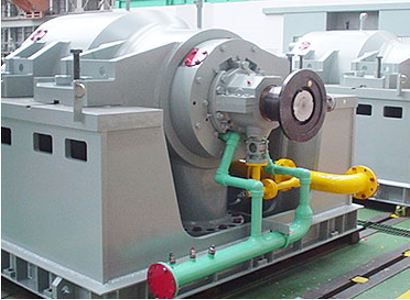
Machine Condition Modeling
Based on 6 weeks sensor data, Dataneuro created condition model of the machine in 3 minutes.
Abnormal Analysis
Dataneuro identified 7 anomaly time stages (5 were before the malfunction) in 1 min. and identified sensors with abnormal changes in each time stage.
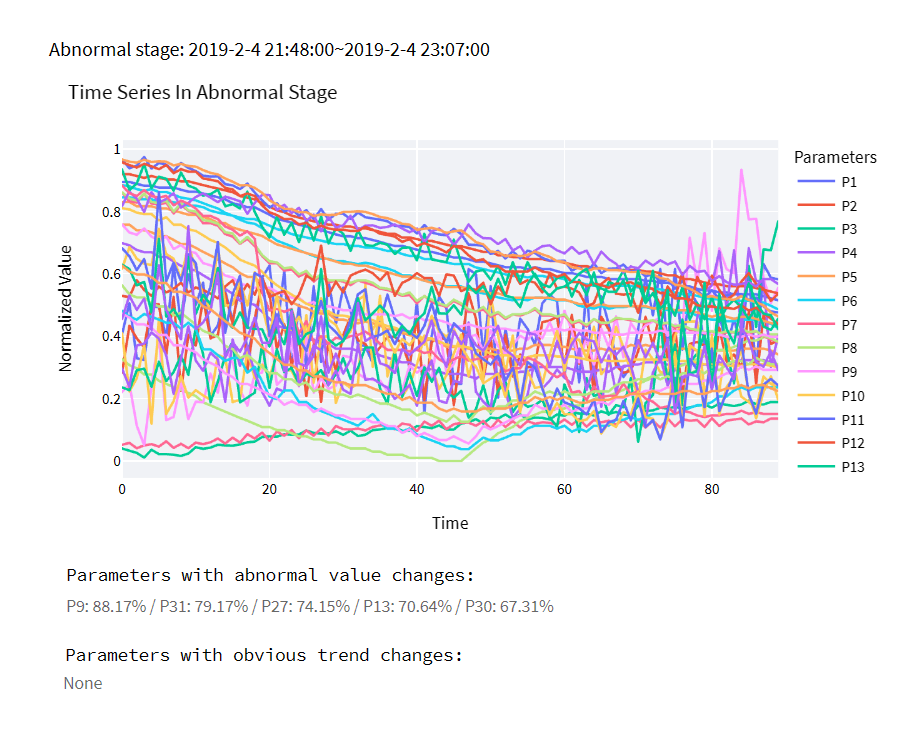
Health Trend Assessment
Dataneuro identified the asset condition trend and pointed out the asset condition was already getting worse about one week before the malfunction.
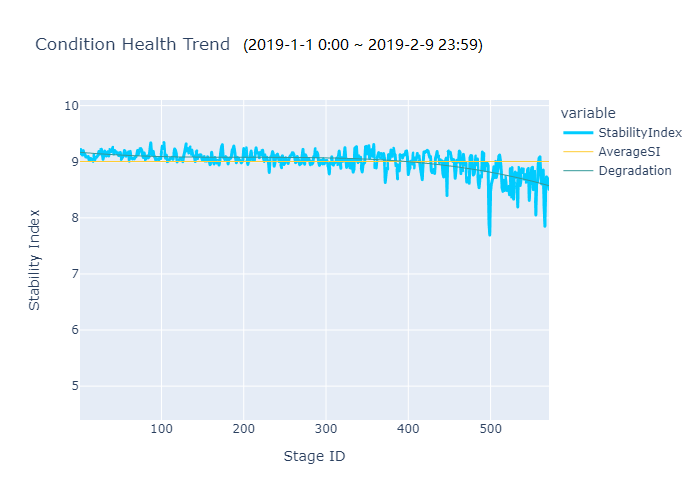
Summary
Dataneuro completed the asset condition assessment in a few minutes without lengthy and costly preparation & learning period and expert involvement. (1 model, 5 min. for modeling + analysis) compares to (n models, hours for modeling + analysis).
"If you never try, you never know."
Please mail to us for arranging a concept demo:
marketing@ingshi.com.cn
FAQ
Predictive maintenance technology uses process data and advanced analytics to predict mechanical failures ahead of time to enable machine owners to make better decisions around the maintenance.
Predictive Maintenance | Preventive Maintenance |
---|---|
Active: It is performed to predict failures that might occur. | Passive: It is performed to prevent assets from unexpected failures. |
It is real-time. | It is done on a regular basis. |
No downtime of machine is required, i.e. one does not need to stop main functions of assets as this maintenance can be performed while assets are performing their regular functions. | One needs to increase downtime of the asset to carry out maintenance, i.e. one needs to stop main functions of assets to carry out maintenance action. |
Maintenance occurs only when potential failures are identified. | Maintenance occurs even if potential failures are not identified. |
This maintenance action is less costly than preventive maintenance as one can simply reduce avoid maintenance that is not necessary and thus reduce maintenance costs. | This maintenance action is more costly than predictive maintenance, as regular maintenance requires more investment. |
It is less time-consuming as in this type of maintenance, one needs to perform inspection and maintenance only when required. | It is more time-consuming because in this type of maintenance one needs to perform inspection and maintenance regularly. |
Cloud plateform or On-premise (Windows) system
Dataneuro XX.NET are B/S systems and can integrate with 3rd party systems (like MOM, EAM, SIS, APM, etc.)via WebService data interfaces.
Yes.
In Dataneuro, user can integrate field expertise with data analysisi results (like data pattern) via labeling correlative data patterns and defining rules of abnormal stage judgement. /p>
Yes. We also deliver the following services to our clients upon request: 1) Machine or process data pre-processing, like data clean, merge or sorting, etc. 2) offline machine condition assessment and report.